Artificial voices sound like us, but they lack fundamental qualities of human speech
Professor of linguistics Emily Bender discusses the limitations of speech powered by LLMs and why it’s fundamentally different from human speech.
Listen 21:03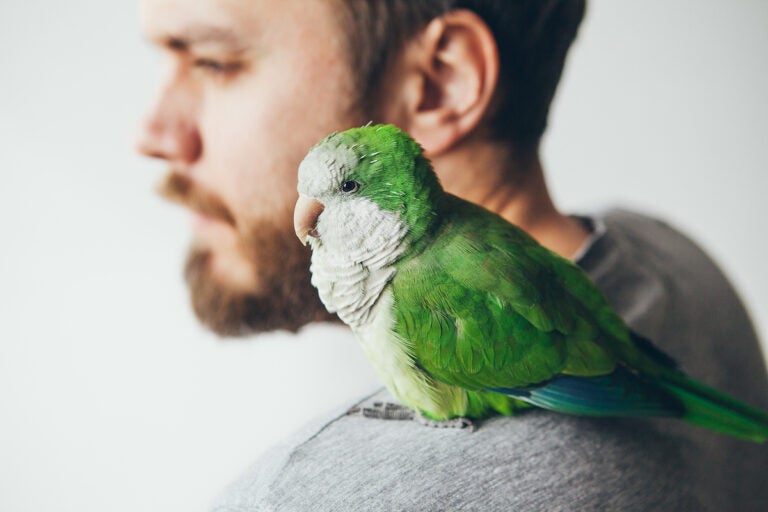
A Quaker parrot, known for its ability to mimic human speech, sits on the shoulder of a young man. (Bigstock/Insonnia)
This story is from The Pulse, a weekly health and science podcast.
Find it on Apple Podcasts, Spotify, or wherever you get your podcasts.
Just a few years ago, it was pretty easy to tell if you were communicating with a human or a bot. But now, voices and text powered by artificial intelligence and large language models (LLMs) have become so good, so real, the difference between them and actual humans is getting blurred.
Human language is one of the key features that makes us unique. It’s how we express ourselves, share, and connect with each other. So what does this presence of AI generated language mean to our existence? And how does it challenge what it means to be human?
Emily Bender, professor of linguistics at the University of Washington, has been critical of some of the hype around LLMs, like ChatGPT, in relation to human speech.
Bender says it’s key to understand that these systems don’t reason or create, they put together information based on probability. She has called them, “stochastic parrots.”
“It is a way to make vivid the notion that these systems are not understanding, having some thoughts, doing some reasoning, and then coming back with an answer,” says Bender. “Instead, they are … stitching together sequences of letters, even, not just words, from their training data in a way that matches the probabilities.”
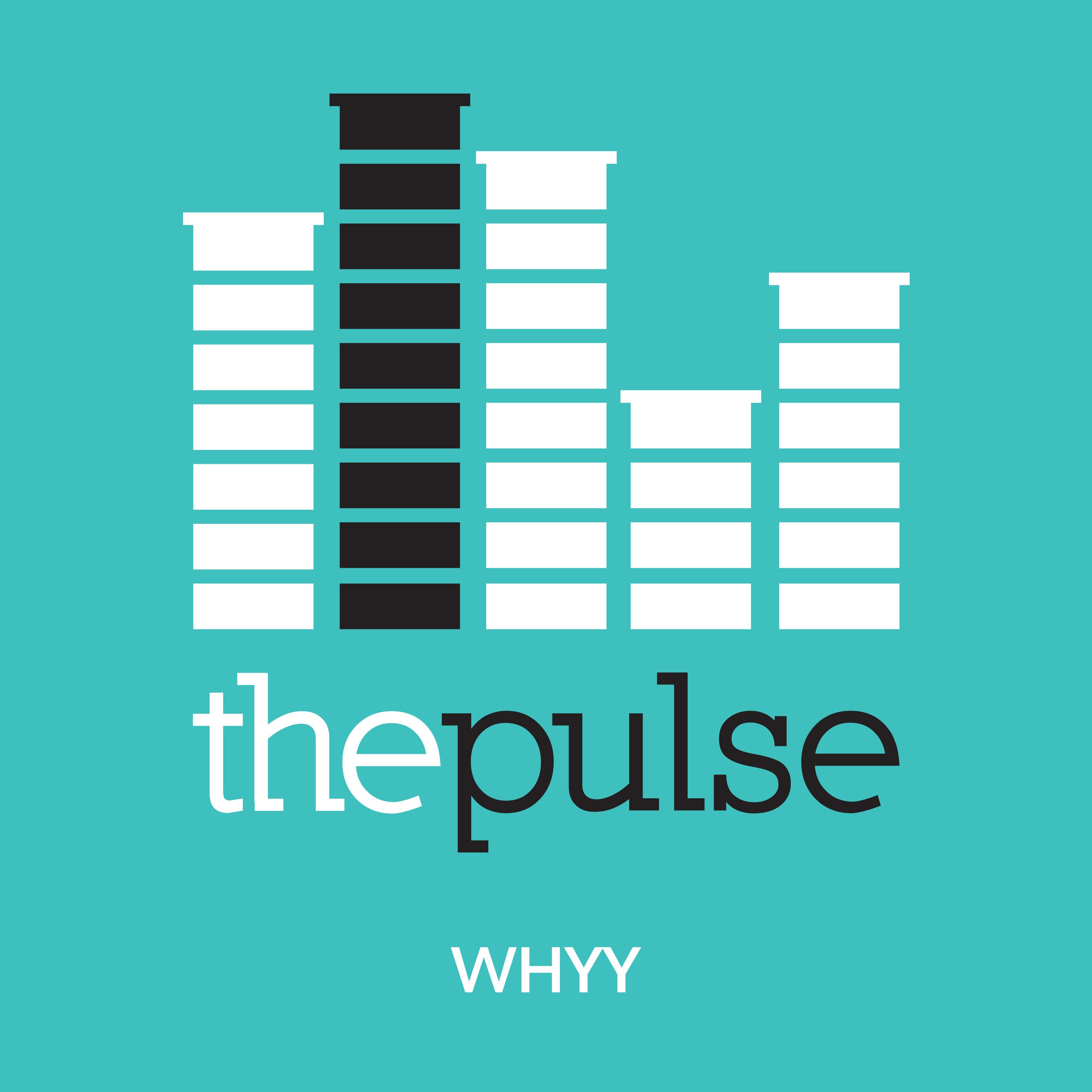
Subscribe to The Pulse
Some leaders in AI, have argued that humans also repeat what they hear from others, making the case that we, too, are stochastic parrots. Bender disagrees.
“It’s true that we get ideas from other people, and we build on them, and we learn phrases from other people, and we reuse them. But when we do that, we’re doing it because they’re meaningful to us. And we’re doing it with communicative intent. And to reduce our experience and our activity to just its observable form so that you can claim that a language model is as good as a human is really to devalue what it means to be human,” Bender said.
Bender makes it her mission to show that while, yes, these machines are producing human-like speech, it is not the same as human communication. She goes more in-depth about the limitations and dangers of AI and LLMs in a conversation with Maiken Scott, host of The Pulse. Listen to the interview using the audio player above.
Interview Highlights:
Where does language get its meaning from?
As a linguist, I see two levels of meaning. On the one hand, there’s what we call the conventional meaning. That is, within a speech community, we have shared knowledge about what a word like book, or cat, or dog, or rainbow, or sleep – you know, it doesn’t have to be just nouns – what these things refer to. And that’s a resource that we all have access to when we speak to each other.
And then the second level is really communicative intent. That is — what it is we are trying to get across when we choose some words, say them in a certain order, in a certain context. And both of those things are meaning. And the only thing a language model has access to is really a shadow of the first one. Because words that mean similar things are going to show up in similar contexts. And that’s all that the language models are modeling.
Even humans struggle with meaning sometimes, especially when we’re reading text messages. It’s difficult to infer the tone…
The fundamental use of language is face to face, co-present communication where we can see each other. We can share the same environment. And we have everything that goes into our inflection, our tone of voice. Are we smiling or not? What you can hear, right? Over the radio, you can hear a smile. But in a text message, you can’t see it. And so we have to do a lot more inference when all we have is words and maybe some emoji compared to what we do when we are face to face.
Why are some chatbots designed to make us think they are human?
There’s all kinds of design choices behind that, right? There’s absolutely no reason that a chatbot should use the “I” pronouns, because there’s no “I” in there, right? But they are designed to do that. And I think part of it goes back to the history of trying to build systems that are familiar and easy to use.
There’s this idea that it’s good for technology to be frictionless. And because we’re used to using language to talk to other people, then if we can just use it the same way to talk to a computer, then the computer will be easy to use. I think that is some of the desire. And I think it’s really misguided because that is a design choice that makes it hard to understand what the system actually can do — and what you can — and should not use it for.
What is the danger here? What is at stake?
So at an individual level, there is enormous chances of misinformation, right? If you have something that is speaking authoritatively and taking on the tone of, you know, a Wikipedia article or a medical explainer or something like that, it is very easy to take that as something that is knowledgeable and then make bad decisions based on incorrect information …
You go one level up and you think sort of about our information ecosystem. People take this information. ‘Hey, ChatGPT told me,’ and then they put it out into the world. And they don’t necessarily say where they got it. And now we have pollution sort of flowing through the information ecosystem.
What are people doing to distinguish their speech from LLMs
I’m intrigued to see what happens as people deliberately use language in more creative ways to distinguish what we’re saying from the output of machines.
… coming up with new metaphors, coming up with, you know, new slang, for example, things that aren’t in the training data. And one of the things that sociolinguists observe is that various kinds of pressure can drive rapid language change. So if you’ve got a community that is stigmatized and marginalized, they might rapidly develop and turn over slang to make sure that their speech is not comprehensible to outsiders.
And so it’s a different kind of a situation, but might lead to similar outcomes, where if we have this homogenizing force of these systems that output the likely next word, and if people start using them as writing assistance, then you’re going to get very similar text and there’s then space for people to distinguish themselves by coming up with a new metaphor, by coining new terms by using words in new ways. And that can be an exciting linguistic moment.
This interview has been edited for length and clarity.
WHYY is your source for fact-based, in-depth journalism and information. As a nonprofit organization, we rely on financial support from readers like you. Please give today.