Transcript: Biased Intelligence (A.I. Nation)
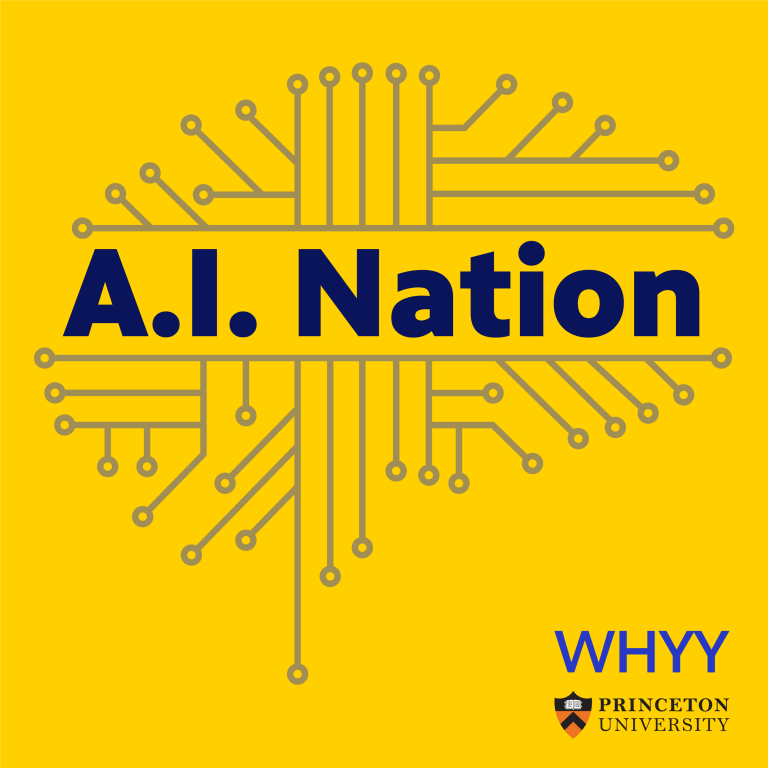
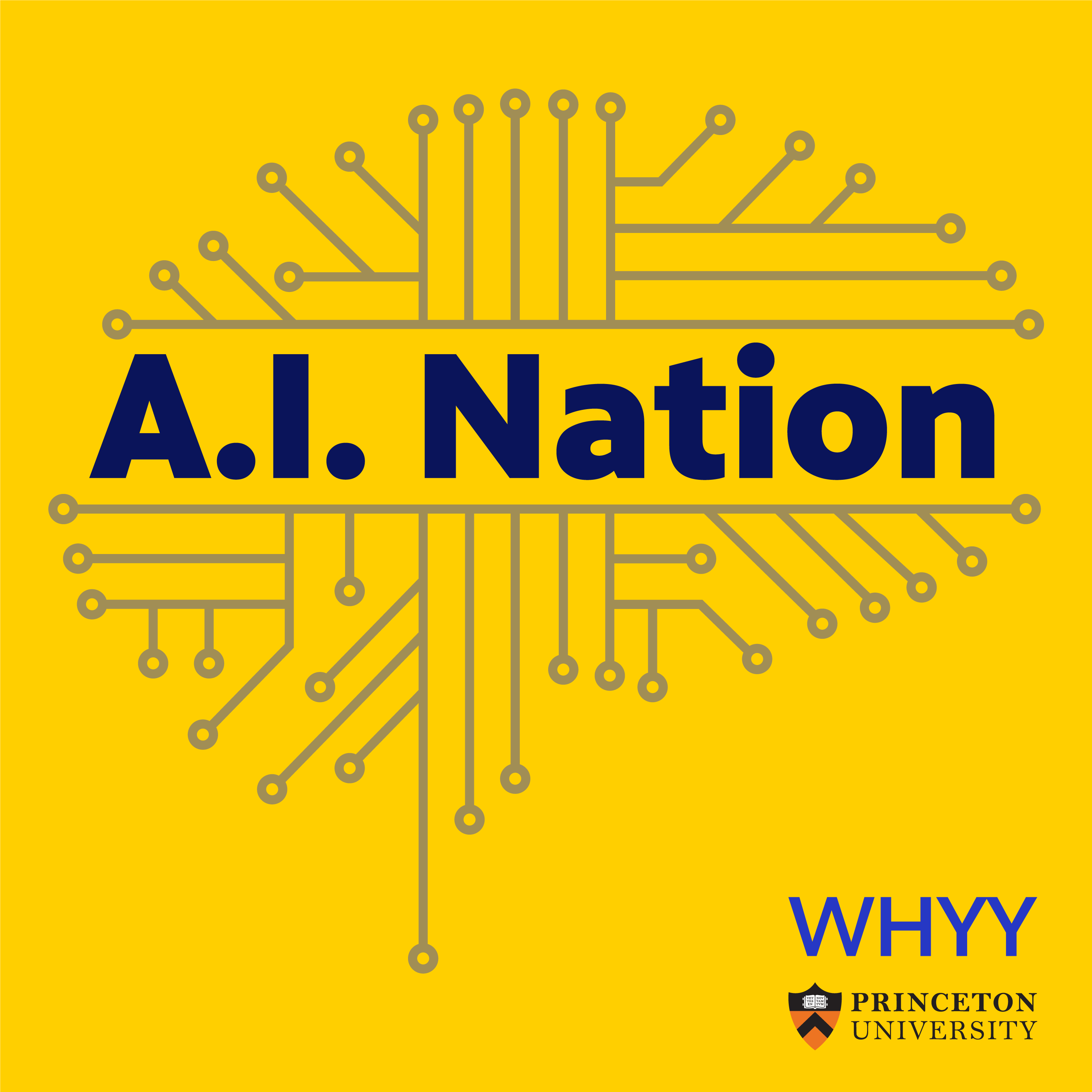
Listen to A.I. Nation
[music]
MALCOLM BURNLEY, HOST: In early 2019, Nijeer Parks learned there was a warrant out for his arrest.
NIJEER PARKS: I got a call from my grandmother. I was actually on my way home, and I got a call from her telling me not to come home at the time. So I’m like, I was kind of startled, ‘Like what do you mean, not come home?’ She was like, ‘Well there’s a lot of police here looking for you.’
MB: Nijeer is 33 and lives in New Jersey. He was afraid that if the police came looking for him at his work, he’d be fired. So he called them up. The person on the phone told him there was a warrant for his arrest, but wouldn’t say what for, and that he should come to the Woodbridge Police Department.
Nijeer lives half an hour away and says he’d never even been to Woodbridge, let alone committed a crime there. So he figured he was just heading over to explain a misunderstanding.
When he arrived, he showed the receptionist his ID.
NP: And while she was talking to me, two officers just walked up and asked me to put my hands behind my back. So I turned around, I’m like, ‘For what?’ They’re like, ‘You’re under arrest.’ ‘I’m under arrest for what? Like I came here to handle, to see what was going on. I didn’t do anything wrong!’
MB: The police started questioning Nijeer and something was said about assaulting a police officer. At this point, Nijeer was panicking.
NP: I grew up in the streets most of my life, so I’ve seen things happen, so I know what kind of things happen when they think you harmed one of their own. If they think you harmed a police officer, they’re going to beat the crap out of you, when they get you.
MB: He says it seemed like they were already convinced he was guilty. Nijeer had prior convictions, which he thought was part of the reason. But they also kept saying this thing.
NP: You know what you did. You know you did it. You know the computer’s not going to lie.
MB: The computer’s not going to lie. What Nijeer didn’t know at the time was that the police had run a photo of a shoplifting subject through facial recognition software. The man was several inches taller than his year. And unlike Nijeer, he had pierced ears. But the facial recognition software said Nijeer was a match.
Nijeer spent 10 days in county jail and was eventually let out on bail. When he got his phone back, he found a picture he’d taken the day of the shoplifting, of a receipt from a Western Union half an hour away from the crime scene. Nijeer sent that picture to his lawyer.
NP: He called me back maybe I think the next day and was like, ‘Yo, that’s the same day, and it’s a couple, it’s only like a 10, 15 minute difference. There’s no way you could have did this and been back up there in that amount of time.’
MB: Woodbridge dropped the charges against Nijeer. Now he’s suing the police, the prosecutor, and the city for false arrest, false imprisonment, and violation of his civil rights.
NP: I do feel like I should be financially taken care of for what I’ve been through. But my main thing is like so it don’t happen to anybody else. The next person might not be lucky and might not have a receipt for where he was at that day.
MB: Nijeer is the third Black man in New Jersey police have arrested based on faulty facial recognition.
[new music]
MB: From WHYY in Philadelphia and Princeton University, this is A.I. Nation, a podcast exploring how humans are handing over more and more control to machines, and what that means for us. I’m Malcolm Burnley.
ED FELTEN, HOST: And I’m Ed Felten, a computer science professor at Princeton University, and I worked on technology and AI policy with the Obama Administration.
MB: We’re going to be talking about bias in AI today. People have this tendency to assume computers are neutral and always right — like those police officers who questioned Nijeer and said, “The computer’s not going to lie.” That assumption is so common it has a name: automation bias.
This very unfunny problem makes me think of kind of a funny scene in The Office, where Michael and Dwight are driving back from a sale and the GPS insists they make a turn.
GPS FROM THE OFFICE: Make a right turn.
DWIGHT FROM THE OFFICE: No wait wait wait wait, no no no it means bear right, up there.
MICHAEL FROM THE OFFICE: No it said right. It said take a right.
MB: Despite the fact that there’s very clearly a lake over there…
DWIGHT: It can’t mean that! There’s a lake there!
MICHAEL: I think it knows where it is going!
DWIGHT: This is the lake!
MICHAEL: The machine knows!
DWIGHT: This is the lake!
MICHAEL: Stop yelling at me!
DWIGHT: No! It’s a lake! There’s no road here!
[splash]
EF: I mean there is this tendency to trust something because it’s high tech. But a lot of times it’s a complicated situation, you’re not sure what’s right, and you don’t really understand how the computer works or how it got to that result, so it’s one of the big challenges in AI is how you know when you should trust it.
And sometimes the stakes are low, and you can just you know live with the consequences one way or the other. But sometimes the stakes are high, right? You end up driving into a lake or you’re making a decision about some patient’s healthcare.
But bottom line is, like everything that’s made by people, if we don’t take care to keep bias out of it, bias will get into it and the results can be unfair.
MB: Take facial recognition technology. It’s now advanced enough that you can use it every day to unlock your phone. But it isn’t flawless.
MB: And so the federal government put out the big kind of landmark study in 2019, I should say, not the government, the National Institute of Standards and Technology. And that was the one that is often cited because it found that identifying Asian-American and African-American faces were up to 100 times more likely to be misidentified, using this facial recognition technology. How accurate is it right now from your perspective?
EF: The answer, unfortunately, is it depends. One of the things it depends on is which commercial facial recognition algorithm is being used. One of the takeaways from the big study that NIST did is that there are really significant differences, both in accuracy and also in the degree of demographic differences. That is, some algorithms have a much more severe problem with being less accurate for people of color than for others. And some other algorithms have less of an issue with that. So it really does depend on the details of what algorithm you’re using and what settings you’re using it and so on.
MB: Bias shows up in all areas of AI. In this episode, we’re going to focus on what happens when this bias shows up in AI used by the police. This is because the consequences can be so huge. Here’s Nijeer again:
NP: Someone asked me, how was it so scary to go to jail, when you’ve already been to jail?
MB: Hm.
[music]
NP: And I told them, any time you walk inside any type of jail, there is no guarantee that you’re going to walk out.
MB [to Ed]: A lot of people hear AI, they think of it as neutral, maybe objective. But there’s a lot of discussion about how bias can make its way into artificial intelligence, into algorithms. Can you explain that aspect —
EF: Sure.
MB: — of how bias might make its way in?
EF: There are a bunch of ways that bias could find its way into the results of an AI algorithm. One of the simple ones is, if you have a data set that the AI is trained from that has more data about certain groups and less data about other groups.
MB: This is one of the things that can go wrong with facial recognition software. Engineers provide the algorithm with a data set to learn from: a bunch of photographs of faces that it analyzes for things like distance between eyes, chin size, things like that. If most of the photos are of white people, the algorithm will be worse at identifying people of color.
EF: Another way bias can get in is if the algorithm’s training data, that is the examples that you give it to learn from, come from the past decisions made by people. So imagine that you’re training a system to make decisions, say, about who gets a loan from a bank. And so you could take all of the past decisions that bank’s loan officers have made about who got loans and who didn’t get loans. And you could train an algorithm to say, ‘Do it like our people have done it in the past.’ The result is that if those past loan officers have been biased, or if the company structured its business in a way that had biased effects in the past, then the algorithm will faithfully reproduce that bias in the future.
MB: That problem — replicating the bias of past decisions — is a particular issue when it comes to something called predictive policing.
EF: I think predictive policing is the main event when it comes to law enforcement and AI.
MB: A lot of AI uses predictive algorithms. They’re part of how autocorrect guesses what it is you actually meant to say in that text. They’re a part of how driverless cars anticipate crashes. And some police departments use them to try to figure out where a crime is likely to happen, or who is likely to commit a crime.
In pretty much everything I read about predictive policing, the movie Minority Report came up.
MINORITY REPORT PREVIEW: The future can be seen. We are arresting individuals who have broken no law. But they will! The fact that you prevent it from happening doesn’t change the fact that it was going to happen. Murder can be stopped!
MB: How is predictive policing and sentencing any different than that dystopian future that was portrayed in Minority Report where people can be arrested for crimes that they are expected to commit in the future? And of course, the caveat is, I know in Minority Report they actually have images of people committing these crimes. And I can’t remember the name of the coggs I think…
EF: Precoggs.
MB: Precoggs, there we go.
ED: The main thing that happens in Minority Report that doesn’t happen today is people getting punished for crimes that they haven’t yet committed but will certainly commit in the future, in the future. We don’t pretend that we can predict in that way. So what predictive policing is doing today is it’s sending the police to certain neighborhoods. It’s changing the tactics that police use, in terms of who do they stop, who do they talk, who do they shine their spotlight of attention on. And those are serious issues because having a lot of police in your neighborhood, having police watch you, stop you, ask you suspicious questions, has a real impact on you. It has an impact on your community.
[music]
MB: I talked to Rashida Richardson about this. She’s a lawyer who researches AI, race, and policing.
RASHIDA RICHARDSON: And I’m a visiting scholar at Rutgers Law School and a senior fellow at the German Marshall Fund.
MB: Rashida’s research was cited recently by 8 member of Congress, including Elizabeth Warren, in a letter sent to the DOJ over concerns about predictive policing. She looked at 13 cities across the country that use predictive policing and she found a problem she calls “dirty data.”
Remember, you have to teach these predictive policing algorithms what to do based on a big data set of things from the past, and that data set might not be as neutral as we think.
For example, predictive policing might recommend you send more police to a neighborhood that’s had a lot of 911 calls. Which makes sense at face value: people call the police to report a crime, right?
RR: The internet culture has sort of meme-ified the Karens of the world. But when you have individuals that call the police on others for non-criminal activity, and that type of data is not corrected, then that can also skew both what looks like the prevalence of crime, but also who is committing crime.
MB: 911 calls don’t necessarily mean crime is happening; they just mean somebody called 911. And we’ve seen big instances in the news of white people calling the police on Black people who were just… being Black in public.
AMY COOPER: There is an African American man. I am in Central Park. He is recording me and threatening myself and my dog.
MB: Other predictive policing algorithms may use arrests in their data sets. But, again, arrests don’t always equal crimes. All of the 13 cities Rashida studied had long histories of Civil Rights violations. Many had patterns of wrongful arrests and police abuse in communities of color.
Essentially, some of the data used in predictive policing is more about policing than crime. And if that policing is biased, the suggestions the algorithm makes will also be biased. Which can make things even worse.
EF: One of the challenges with predictive policing in particular is a kind of feedback loop that happens, that the data the police have reflects where they’ve been. And so the risk is that they will decide they should go back to the places they’ve been before, and maybe even that they should go back there even more intensively than they did before because, look, there seems to be more to be more sort of petty crime happening in these places. And it’s not because necessarily there’s more petty crime happening in those places. It’s because they’re more likely to see it. They’re more likely to encounter someone who complains about it. And therefore their statistics are biased by where they’ve been in the past. Effectively, over-policing causes more over-policing.
[music]
MB: That could mean more police in your neighborhood or more police contacting you specifically. Rashida told me about a program like that in Chicago called the Strategic Subject List.
RR: They were attempting to predict individuals that were likely to be either a victim or a perpetrator of violent crime or gun violence.
MB: The Chicago police would assign people a score, to predict if they were high risk or low risk. Arrests for some crimes, including drugs, factored into somebody’s number. So did their age when they were arrested.
RR: One factor that could result in someone being included on the list is the number of times the individual was a victim of a shooting. So if you’re someone that’s in an area that has had a lot of gun violence and your name was taken down in a police report, that could put you on the list. Then let’s say there were more shootings in your neighborhood, and that’s a factor that counts under trends in criminal activity. Your number could rise simply because of where you live.
MB: Somebody with a high score would get notified that they were on this list, and that the police thought they were more likely to either commit a crime or be a victim of a crime. Rashida says the idea was to connect people on the list with resources.
RR: But research about the actual use found that this is not what actually happened in practice.
MB: Officers didn’t get much training on what to do with high scores. More often than not, they would end up arresting people on the list, and that would sometimes make their score higher.
More than half of young Black men in Chicago ended up on the list.
Rashida says that’s partly because a lot of the factors used to come up with somebody’s score are intertwined with systemic racism.
RR: The problem with a lot of these systems is they’re essentially suggesting that someone will engage or is likely to engage in criminal activity based on where they live or who they know, which in many cities in the United States is a proxy for race because of how segregated society is.
MB: The Office of Inspector General for the city looked into the program and concluded it had probably led to more illegal stops. So Chicago shut it down.
I asked Rashida how common this kind of predictive policing is across the country.
RR: We don’t actually know how prevalent predictive policing is. We know that it’s been used by some of the larger police departments and some small and mid-sized police departments have used the system. But we both don’t have a head count for how many systems have been used over time or how many systems are currently in use.
[music]
MB: So, Ed, is this true?
EF: It is true. The rules are different everywhere. There’s no centralized database of which systems are used where, or how often, or what the results are. We’re really in the dark.
MB: So some towns may use AI in ways that others ban, or some towns may be using it extensively, while others aren’t using it at all?
EF: Sure, some are doing this really well, others are not. But there’s not sort of a unified picture, and there’s not much of a unified strategy.
MB: And so regulation in that sense can only kind of have a limited effect. It sounds like.
EF: Well, first we need to get better transparency into what’s happening, and then I think we can decide what it is that we need to do and whether regulation’s needed or what kind of regulation.
MB: When we come back:
ROBERT CHEETHAM: We felt like technology could improve both public safety and social justice.
MB: Is it possible to create an ethical predictive policing system? I’m Malcolm Burnley.
EF: And I’m Ed Felten.
MB: And this is A.I. Nation.
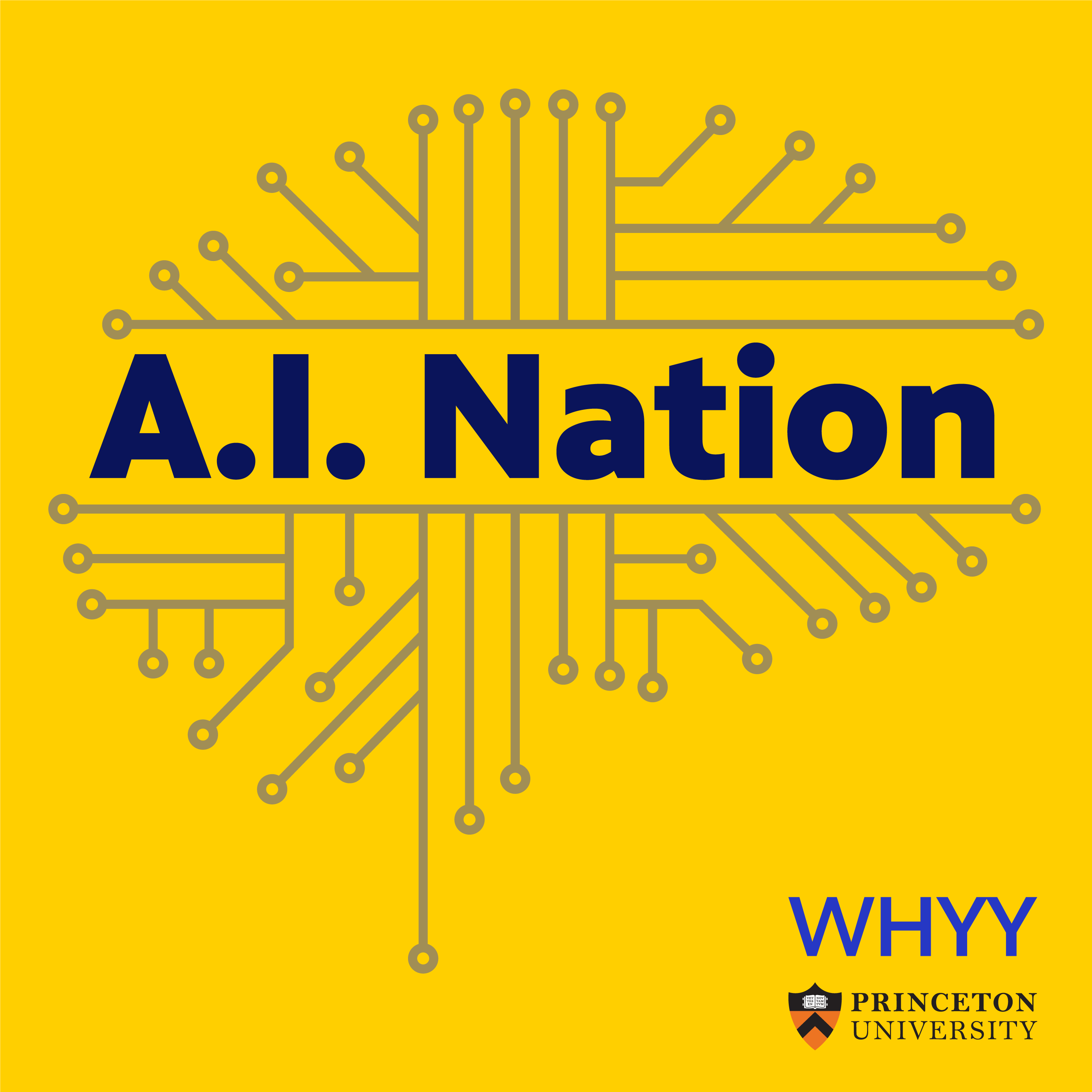
Listen to A.I. Nation
[music]
MALCOLM BURNLEY, HOST: Welcome back to A.I. Nation. I’m Malcolm Burnley.
ED FELTEN, HOST: And I’m Ed Felten.
MB: In 1994, Robert Cheetham designed one of these early predictive police systems we’ve been talking about, but, to be fair, he’d rather his technology be called “crime forecasting.”
ROBERT CHEETHAM: We’re not particularly fans of the term predictive policing. I continue to feel like that’s not a very descriptive term and implies that we are actually predicting crime events. I would say it’s more like the equivalent of a low quality weather forecast. You can get a percent chance that an event may occur in a particular location, but that’s about it.
MB: Robert was working for the Philadelphia Police Department, just as they were starting to map out crime analysis, and he developed a crime spike detector called Hunchlab.
Every night, computers would analyze crimes across the city. And if the computers found outliers, they’d automatically email the police captain with a map, and some additional information. The police captains might then decide to send more police over to an area with increased crime.
Robert eventually left the police department and founded a data analysis company called Azavea, but he still had Hunchlab in his head. Since his time with the police, the technology had evolved. People were using crime data not just to understand the past, but also to try and understand the future.
A lot of the companies working on this tech were doing it to make money. But Azavea was a B corporation — still for profit, but with an obligation to think about the public good. What could a company like theirs do differently with a more advanced Hunchlab?
RC: From the very start we understood it would have the potential to be controversial and would be challenging. I think the simplest thing would have been not to pursue it, but we felt like there was an opportunity to create something that would both help police departments and help communities be safer places.
MB: Robert and the Azavea team felt like it was part of their mission to use technology to improve public safety. If they could help stop somebody from getting assaulted or murdered — well, that was helping society.
RC: Second, we felt like in the United States in particular, there are far too many people arrested, far too many people go to jail.
MB: They thought the criminal justice system was biased and sometimes destructive, and they thought they could use crime forecasting to help. They wanted to offer a product that was more ethical than the ones out there, so that police would have a better option.
So, they got to work. One of the first choices they made? No person-based prediction. They didn’t want to be in the business of scoring somebody, for example. They’d only offer software that forecasted places where crime might occur.
RC: So we made a early commitment to not use arrest data, which is fundamentally data measuring what the police department does, not measuring what happens in a community. We wouldn’t use social media or any other information around criminal backgrounds.
MB: Robert says Hunchlab relied on the least-biased data it could.
[music]
MB: The company used publicly available data about things like street lighting and school schedules. They did include data about 911 calls, but they tried to build in another layer to avoid bias there.
RC: We use data that was a step after 911 data. So after a police department responds to a 911 call, one or more police officers are sent out to respond to that. And then that data gets encoded as an incident or an event with an event classification associated with it.
MB: They’d only include a 911 call in their data set if police later logged it as a serious crime that hurt the community — so robberies, homicides, assaults, thefts.
And Azavea tried to interrupt that feedback loop we heard about earlier, where over-policing leads to more over-policing.
One of the ways they attempted to do that was by tracking officers’ phones. They found studies showing an officer’s presence in a neighborhood is only helpful in deterring crime for 15 minutes. So the software still directed officers to areas where they forecasted a crime could happen — but, after 15 minutes, their phone would ping and let them know it was time to move on.
One of the most interesting things I thought Azavea did was around transparency. They really wanted people to be able to understand how Hunchlab was being used in their communities. They offered police departments a discount if they let the Hunchlab team come in and explain it to the public.
They wanted Hunchlab to be simple to explain. And one of the ways they did that was by rejecting machine learning — that big advance in AI we’ve been talking about.
RC: The statistical models that end up being built are pretty hard to actually explain. The learning process ends up resulting in a model that it’s not entirely clear how it came to the conclusion that it did. We got some good results from it, but we decided not to use it in Hunchlab because we couldn’t explain how it gets to the conclusions that it did.
MB: Azavea sold Hunchlab to about a dozen big cities. In 2018, the company decided the product was growing faster than they could keep up with. They sold Hunchlab to another company, ShotSpotter. It’s available today under the name “ShotSpotter Connect.”
I asked Rashida Richardson, the lawyer studying predictive policing, if she thinks all of this is enough — if it’s possible, taking steps like Robert and Azavea did, to do ethical predictive policing.
RASHIDA RICHARDSON: I don’t think there’s a way to use predictive policing in a way that’s equitable or aligned with principles of justice as they are currently developed, and with the current data available. It’s not to say that somewhere down the line you can’t use police data or even predictive analytics to solve some type of policing problem. I just don’t think it can be used in an equitable way to predict crime or criminal activity.
MB: She says race is too enmeshed in data sources to create a system that doesn’t have bias in it — and that a system like that can’t be fair.
[music]
MB: I asked Ed what he makes of this, too.
EF: Can you eliminate bias entirely? No, I don’t think so. I don’t think so. Can you do better than you would do using the old system? Probably, yes. But it would be really hard. And you have to learn and develop that over time
MB: What Ed, Rashida, and Robert agree on is that there is an element of bias that is unavoidable here. The question remains: What do you do about that bias?
Robert thinks the best thing to do is try your hardest to get to as close to perfect as possible.
RC: I think the alternative is to simply not engage and not take on challenging work.
MB: What that means now is that we’re in a kind of trial period — a trial period that has lives in the balance.
You have companies like Robert’s that are trying to design a more ethical software. You have other companies that… aren’t trying so hard. You have towns and cities across the country buying these products, or making their own. Sometimes the public knows, and sometimes they don’t.
Lately, awareness around predictive policing and bias has been going up. More research has come out in the last few years, and it’s gotten more attention from the media. Some places have banned its use. That includes Santa Cruz, California which was one of the first cities to start using this technology in the first place.
But Rashida said she’s also seeing an increase in reliance on this type of technology.
RR: What I found really troubling is last year, during the rise in protest around racial justice and police brutality — at the same time there seemed to be a public awakening about the problems of policing, I saw many police officials almost double down on the need for predictive policing and other data-driven technologies.
MB: Police departments trying to stamp out bias and racism are turning to systems like these, thinking they’re less biased. Which makes me think about Nijeer again.
NIJEER PARKS: You know the computer’s not going to lie. You know what you did. You know you did it. You know it’s you.
MB: The way things stand now, there isn’t much incentive for police departments to be reflective about how they’re using AI like predictive policing or facial recognition.
A lot of the time, departments get the money to buy or create something like Hunchlab through federal grants. But those grants don’t include funding for evaluating the programs to see how they’re working. Rashida says that’s something we could change.
RR: You can create some type of safeguards or at least regulatory constraints by simply requiring government to do a little bit more due diligence upfront before they purchase these technologies, as well as ongoing reviews around its use.
MB: Robert would like to see some regulations around transparency, too.
RC: I would propose civic algorithm or civic software review boards. There’s no reason we couldn’t have public bodies that evaluate software and algorithms and and and look for bias and look for ways in which they could potentially harm a community.
MB: Ed, we’ve been talking about this moment, where we need to make big decisions about what we want AI to be involved with in our lives and in our communities. This feels like a big example where it’s already here, and we’re instead asking if we’re okay with what we’ve done.
EF: It’s being used in a lot of places, and we’re kind of trying to catch up. But that’s true in a lot of areas, not just policing. AI has crept in and we’ve been kind of slow to recognize it and kind of slow to grapple with the questions that it raises.
[music]
MB: Okay, I’d like to introduce you to one more person.
RIA KALLURI: I’m Ria Kalluri, or Pratyusha Ria Kalluri, but I go by Ria.
MB: Ria is a PhD student at Stanford studying computer science and AI, and is founder of the Radical AI Research Community, a group that looks at how AI shifts power in society.
Ria pushes back against the concept of de-biasing — that is, finding the flaws in AI that make it biased and just trying our best to fix those flaws.
RK: Bias is actually not the right framing of this, like bias sort of makes it feel like a bug that needs to be fixed, when it’s actually like a symptom of these much deeper problems with the way these systems are being built.
MB: A feature, not a bug.
RK: It’s really apt that policing and AI is, you know, being tied together finally in our conversations because there is such a deep relationship between these two things, right? So the, ‘It’s just bias in AI; that’s the only problem’ [argument] is a lot like the ‘Oh, it’s just a few bad apples’ [argument], right? It’s just a few problems in policing or a few people. It’s not just a few biases or a few bad apples. The system was designed to surveil people, to punish people.
MB: If racist AI happens because of systemic racism — Ria says maybe the AI shouldn’t be the focal point of the conversation.
RK: Yeah, I think that machine learning in some ways is, like, fundamentally conservative. Because machine learning, it’s about taking data from the past, learning the patterns in that data, and then applying them to the present.
MB: Machine learning itself is biased towards the status quo. So how do we create AI that doesn’t replicate bad patterns? Ria turned to sci fi.
[music]
MB: This is something we see a lot in the creation of AI, like those Alexa engineers that were inspired by the Star Trek computer. The idea is: you have to imagine something before you can make it a reality.
So Ria created a workshop where people could think about that possible future, and brought people together, often from marginalized communities, to imagine, write and make art about it, and then they’d merge all of their art together in a giant mural.
MB [to Ria]: What are some of the futures that people did imagine?
RK: There are a lot of beautiful dreams about the role of A.I. as a connective force, where AI can sort of connect people in this relationship that kind of mimics mutual aid.
MB: A computer that connects people to one another, so they can share resources with their community.
RK: Another really important dream that’s come up is lots of folks say: Well, I did this whole activity. And my very sincere answer, as I was writing, as I was painting, all of this stuff, is my dream future had no AI, right?
MB: [laughs] Right.
RK: Like, I was a Luddite. Everything was not technological!
MB: I asked Ria if policing ever comes up when people are dreaming of these AI futures.
RK: If anything, I think that when it comes up is really that first 5, 10 minutes of the workshop when we’ve gotten into the practice of asking folks like, ‘Hey, what do you need to say or write down about your biggest hang ups, your biggest fears about this?’ And I think that’s honestly like the biggest place that anything about policing would come up is, like, ‘Oh, man, the fears around this are so deep that it’s hard to even imagine another type of AI.’
MB: Ria thinks workshops like this are just one part of how we should be thinking about AI in the future… But Ed, I’m curious what your reaction is to hearing about their workshops and their take on bias and AI?
EF: Well, I kind of wish I’d been there, you know? it’s fun and important to think about what kind of world we want to live in. But I’m also an engineer, so I’m trying to think about how I can use the tools I have to solve problems and what we can do sort of realistically and step by step to try to make things better.
MB: If you were there Ed, what do you think you would have contributed?
EF: Well, I think I would have asked questions about how we can use this technology to better understand some of the issues like bias. AI is a really powerful tool for finding patterns. And so we should turn that on to questions of bias and ask, How can we organize things to try to reduce some of the problems that happen when police and citizens are encountering each other sometimes in highly emotional situations?
You know, people have studied things like what are the factors that are associated with excessive violence complaints against police officers. And some of them are the ones that you might expect — an officer who has a history of violent behavior and so on. But other ones have to do with things like working too many shifts over too short a period of time, or a person who has responded to a call where something traumatic happened, like a suicide or domestic violence involving children. After that, officers, it turns out, are more prone to overreact, just as anyone would. And so police departments can use this data to schedule, for example, to schedule officer shifts in a way that gives that person who is pushed to the edge a little bit of extra time.
MB: Right. I think often people’s initial reaction is how police will use AI against them or to surveil them, right? But what you’re describing is also how departments can use AI for accountability or internally how to make them better.
EF: Right. And also to use it to serve the public in ways other than the crime fighting function of police, because police end up dealing with a lot of social and personal problems that people have, that are not about preventing crime. And if we can help them do that better and more effectively, if they can be better helpers for people when they’re needed to do that, that’s a big win as well. And AI has a lot to contribute there.
[music]
On our next episode: the AI in your pocket that’s changed the fabric of our country — how social media companies, their algorithms, and their priorities are polarizing the country.
A.I. Nation is produced by me, Malcolm Burnley, and Alex Stern. My co-host is Ed Felten. We have editing help from Katie Colaneri and John Sheehan. This podcast is a production of WHYY and Princeton University.