Transcript: Hello, World (A.I. Nation)
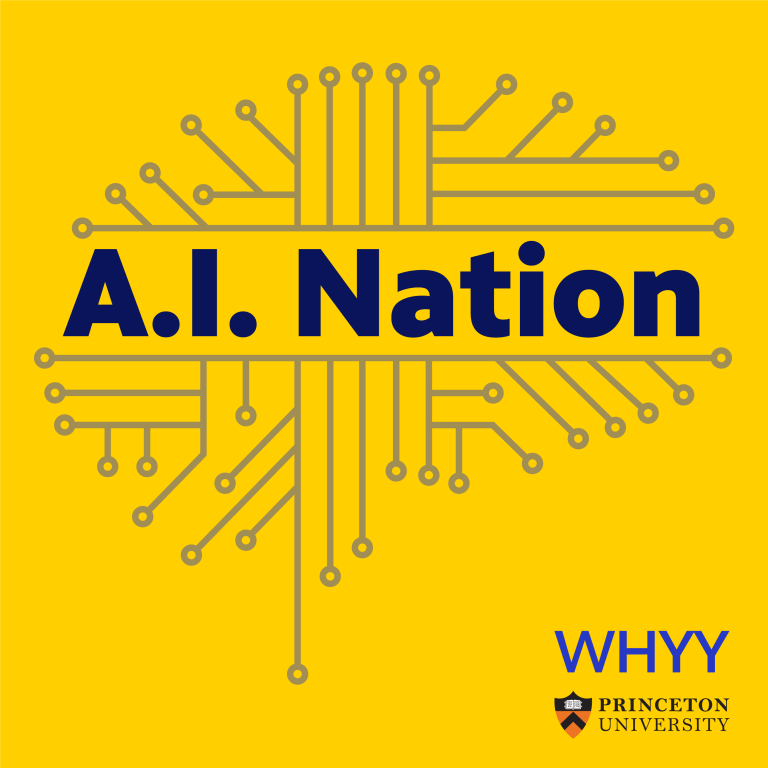
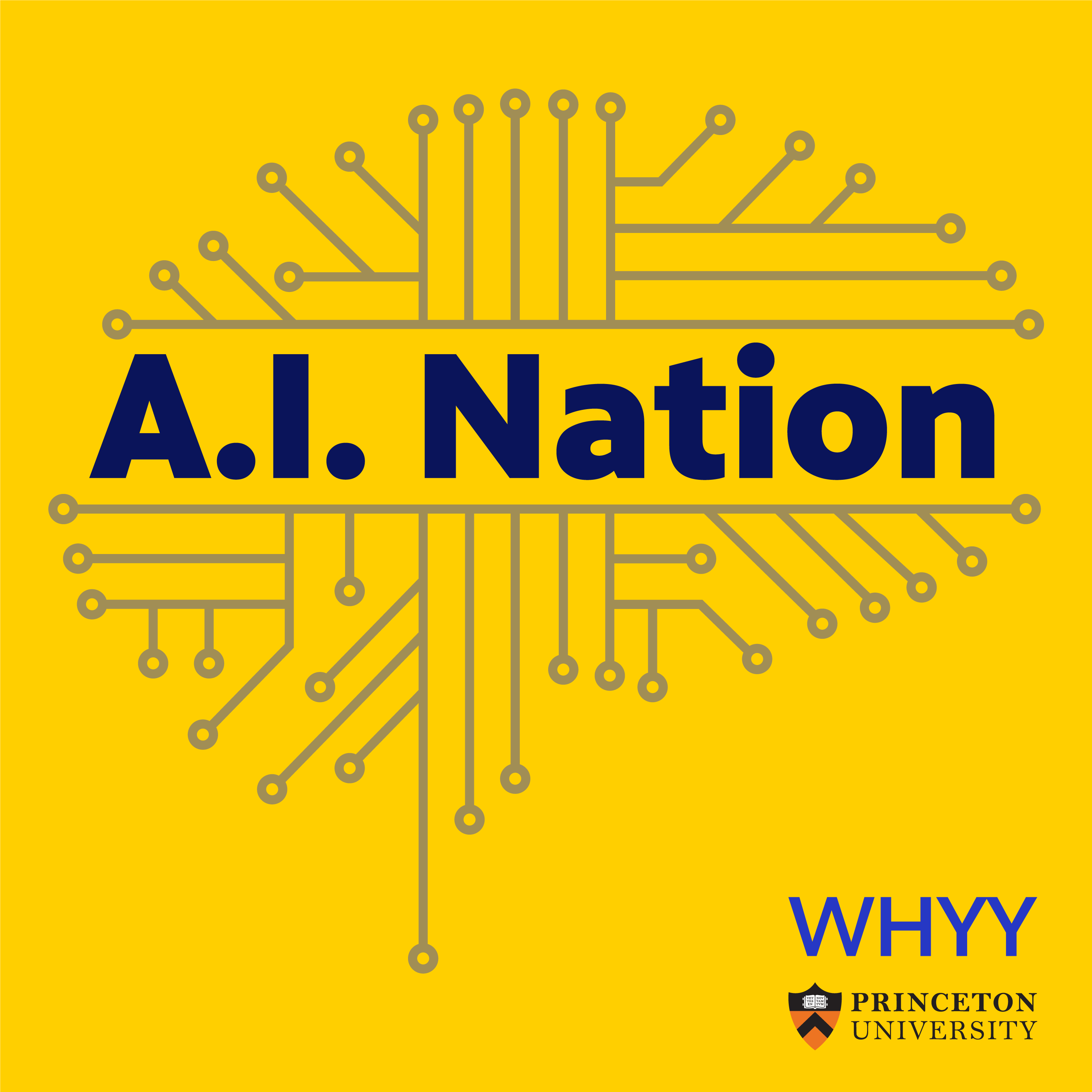
Listen to A.I. Nation
[low music]
ED FELTEN, HOST: I think if you put AI together with the computer revolution and the internet revolution, we’re looking at a major turning point in our history.
[beat drops]
MALCOLM BURNLEY, HOST: Artificial intelligence isn’t science fiction. It’s very real, and getting faster, more powerful, and more widespread every day. In fact, in obvious and invisible ways, AI is humming in the background of all the major events, trends, and stories in our modern lives — like the tools scientists and doctors developed to fight COVID-19.
TAYAB WASEEM: There’s no reason we can’t use big data and AI for not only just the next pandemic but for diseases we have now.
MB: Like the algorithms spreading virulent and polarizing content on social media.
NINA JANKOWICZ: Facebook prioritizes content that’s emotional. Often I say the most engaging content on Facebook is the most enraging content.
MB: Like AI in law enforcement, which is already happening with little transparency.
RASHIDA RICHARDSON:We’re experimenting on live human populations, and often without any form of public consultation or awareness.
MB: From WHYY in Philadelphia and Princeton University, this is A.I. Nation, a podcast exploring how humans are handing over more and more control to machines, and what that means for us.
I’m Malcolm Burnley. I’m a journalist, but let me be clear: I’m not a tech reporter. I’m not an engineer either. Luckily, this where my co-host Ed comes in.
EF: I’m Ed Felten. I’m a computer science professor at Princeton University and I’ve worked on AI for a long time. And Malcolm, you have no background in AI?
MB: Uh no, I don’t, and to be totally honest with you Ed, I’m definitely something of a luddite.
EF: Well even tech people don’t always love the technology.
MB: If I may add, Ed also worked in the White House.
EF: Yeah I worked in the Obama administration, as their Deputy Chief Technology Officer. One of the big things I did is I quarterbacked the policy initiatives around AI and machine learning.
MB: So you were the guy basically standing between humanity and the machines.
EF: I wouldn’t go that far. But I was part of the team that was trying to figure out what to do about things like killer robots, and make a plan. When I was in the White House, I had in the corner of my whiteboard this scoreboard for the administration, which had the number of times we’d broken the internet and the number of times of robot apocalypse that happened. And thankfully they were both zero all the way to the end.
MB: [laughs] But the fact that you did have a category of robot apocalypses implies that it could happen.
EF: A lot of people are trying to stop it from happening, of course.
MB: And as much as technology isn’t my thing, artificial intelligence is a personal fascination of mine. And I love sci-fi stories of man versus machine — although, I’ll admit, some versions of AI in pop culture like The Terminator…
CLIP FROM TERMINATOR 2: The war against the machines. [glass shatters]
MB: Hal in 2001…
CLIP FROM 2001: A SPACE ODYSSEY: I know that you and Frank were planning to disconnect me.
MB: Or the creepy kid in AI…
CLIP FROM A.I. ARTIFICIAL INTELLIGENCE: Is this the place they make you real?
MB: …can get pretty dark and dystopian.
[music]
MB: Growing up, I spent every New Year’s Eve watching Twilight Zone marathons with my dad – the original series with Rod Serling. There was this one episode that I remember so clearly, and it’s about this guy, James Corry, who is serving a 50 year prison sentence on an asteroid 9 million miles from earth, a kind of futuristic solitary confinement.
JAMES CORRY FROM THE TWILIGHT ZONE: I can’t stand this loneliness one more day. Not one more day!
MB: But Corry’s sanity is restored when he gets some company: a robot woman sent to him by the authorities.
ALICIA FROM THE TWILIGHT ZONE: [in robot voice] My name’s Alicia. What’s your name?
JC: Get out of here! Get out of here!
MB: At first he rejects her. But soon enough, they start playing chess, spending time together. And then, he’s not even lonely!
But then, like a lot of Twilight Zone episodes, it ends with an abrupt, cruel turn. Corry gets pardoned, but he’s not allowed to take Alicia with him.
ALICIA: Corry?
JC: No! No!
[A gun goes off.]
MB: The episode ends with a steaming bullet hole in her robot carcass.
ALICIA: [slower and slower, breaking down] Corry … Corry … Corry…
MB: Ed was more into computers than sci-fi growing up, but he says, from what he’s seen, this is something we do a lot: humanizing, anthropomorphizing AI.
EF: I mean, what’s interesting, I think about old sci-fi about AI is that, in terms of the nature of AI and what it’s like, I don’t think it was super accurate. There was kind of this vision of sort of mechanical people.
MB: In some ways, we can train AI to accomplish tasks like us. But operating on its own, it doesn’t always act like a human would. There’s an element of unpredictability, even in the best AI systems.
EF: And this is one of the most important things to understand about AI is that, if it is intelligent — and you can have a whole philosophical debate about are machines really intelligent — but if we’re going to think of them as intelligent, they’re intelligent in a different way than people are.
I think it’s useful sometimes to think of it as like an alien intelligence. So if aliens landed on Earth and their brains work in a different way than ours do — or whatever they have instead of brains — it might be hard for us to understand them. It might be hard for us to predict what they’re going to do. And their intuitions, their blind spots, and types of errors they make, things they get wrong or fail to see, would be very different from us. And the same is true for AI. There are things that seem obvious to us which might be really hard for an AI system to get right. But at the same time, if the AIs had opinions, they would say, why are those humans unable to see in this giant sea of data there’s a clear correlation between this thing and that thing?
MB: For example, take the way AI interprets language. Natural language processing is a huge part of the field. Natural language means human language, as opposed to computer code. And the more a computer can talk to us like a human, the more we can operate seamlessly together.
We’ve got a story for you about the leaps and bounds natural language processing has made in the last few decades. It starts in the mid 1990s — with a prank.
[Jurassic Park theme plays]
The Jurassic Park series was a major hit, and the world had just met Dr. Ian Malcolm, played, of course, by Jeff Goldblum.
CLIP FROM JURASSIC PARK: [Ian Malcolm’s weird and long laugh]
JOHN HAMMOND FROM JURASSIC PARK: You’ll have to get used to Dr. Malcolm. He suffers from a deplorable excess of personality, especially for a mathematician.
IAN MALCOLM FROM JURASSIC PARK: Chaotician, chaotician, actually…
MB: Dr. Ian Malcolm was enormously popular and the character returned in both the sequel novel and film, The Lost World. The novel The Lost World opens with Malcolm giving a lecture on chaos theory at the Santa Fe Institute. And at that time, Melanie Mitchell, a real scientist and researcher, was working at the real Santa Fe Institute.
MELANIE MITCHELL: Santa Fe Institute was actually known for people working in chaos theory
MB: The scientists at the Sante Fe Institute, the real life ones, were pretty sure the character was based on one of their real life colleagues, Stuart Kaufman.
MM: Stuart Kauffman, who coined the term “life at the edge of chaos.” And Dr. Malcolm’s speech very much resembled things that Stuart Kauffman would say.
MB: And around that time, the Sante Fe Institute library started getting requests from outside the Institute for the character Dr. Ian Malcolm’s papers. Which was pretty funny. So some of the postdocs decided they were going to create a fake faculty profile for Ian Malcolm on the Institute’s website.
MM: They were very good computer programmers and also science fiction nerds.
MB: They took it a step further and created fake academic paper titles. The easy thing would have been to just make up the titles, but not these postdocs…
MM: Oh, and I also should say that when you’re a postdoc, you have time on your hands. [laughs]
MB: They created a computer program that could generate text, and they fed it a bunch of Stuart Kaufman’s real papers to analyze. The program, which they called Stu-bot, started analyzing the patterns of how Kauffman wrote. It did that by calculating the probabilities of the relationships between the words he used.
EF: That was pretty hard to do in the 90s. Just to put a few words together and make it sound sort of coherent and sciencey? That was about as much as you could do with the models of that day.
MB: So, for the first one, the postdocs picked the starting word “simulating.”
MM: The computer program that was doing this language modeling picked the next word “the,” and then it looked at “the” and picked the next word “organization.” It looked at “organization” and chose the next word “of.” So these are all using these probabilities. And the whole title ended up being “Simulating the Organization of Structures Defies Expectation.” [laughs]
MB: [laughs] And could you give just a couple of other examples of what the titles sounded like?
MM: Yeah, here’s another: “Combinatorial Considerations in the Organization of Awareness: A Treatise.” [laughs]
MB: Melanie reassured me: it’s not just that these are above my head. These paper titles are pure scientific nonsense, but they sound like they could be real!
The Sante Fe Institute was not impressed, and a couple of months later, to the great disappointment of Melanie and the nerdy postdocs, they took Ian Malcolm’s faculty page down.
But what the postdocs were playing around with — language models, and the way computers interact with human language — is one of the major areas AI researchers have been hacking away at for decades.
Melanie, who still studies language processing, explains why its so important to AI:
MM: Language is so fundamental to our lives and how we communicate. And we want to communicate with computers using our natural language, rather than these artificial programming languages or these sort of quasi-linguistic queries that we give them. And that’s been the goal of the field ever since it started.
Interestingly, a lot of people who are AI researchers now, when they were kids, they watched Star Trek, the TV series and one of the things that was in the series was a computer that people talked to in natural language, and it gave the right response, you know, all the time.
[Star Trek theme]
JEAN-LUC PICARD FROM STAR TREK: Computer, estimate the time from this location to Star Base 4-1-6 at Warp 9.
BEVERLY CRUSHER FROM STAR TREK: Computer, are you familiar with the inhabitants of Tau Alpha C?
COMPUTER FROM STAR TREK: Affirmative.
DEANNA TROI FROM STAR TREK: Computer? Where is Lieutenant Warf?
COMPUTER FROM STAR TREK: Lieutenant Warf is in Ensign Calloway’s quarters.
MM: And that was people have always cited that as being extremely influential in their A.I. research. So that’s kind of the goal they want.
MB: We’re not quite there yet with tech like with voice assistants, but Amazon’s Senior Vice President of Devices has called the Star Trek computer Alexa’s North Star. Alexa, engage!
ALEXA VOICE: Aye, aye, captain.
MB: And that makes sense. When we imagine artificial intelligence, we tend to imagine creatures like us, with similar capabilities, who we can communicate with.
EF: You know we just don’t have a way of talking about complex, intelligent-like behavior that doesn’t use the same language we use to talk about each other.
[music]
MB: One of the first times we projected humanity onto a computer happened in the mid 1960s, with Eliza. Eliza was the first chatbot, created by scientists at MIT.
MM: Eliza was meant to be a psychotherapist.
MB: She was programmed with a bunch of typical therapist templates, and she could use them to engage people in a kind of therapy session.
There are versions of Eliza up online still. I gave one a spin. I asked Ed to read Eliza’s part.
[typing sounds]
MB: Hi Eliza.
EF (AS ELIZA): Hello, I am Eliza. How are you today? What would you like to discuss?
MB: [typing] I’m having problems with my mother.
EF (AS ELIZA): How long have you been having problems with your mother?
MB: Hm. It’s complicated. She’s been really mean.
EF (AS ELIZA): I’m not sure I understand you fully.
MB: My mother has been overbearing. [typing continues]
EF (AS ELIZA): I see.
MB: I think that she treats me like a child.
EF (AS ELIZA): But are you not sure that she treats you like a child?
MB: (For the record, this was a fake example… Love you, Mom!)
Eliza is pretty primitive technology, and she doesn’t always make perfect sense, or respond the way a real therapist would.
EF: It pretty much repeats back to you what you said, in the form of a question. And it knows a little bit about parts of speech. It will change “is” to “are” and “are” to “is” and flipping the perspective from you to it and so on. But really it’s fairly simple pattern matching. What’s really striking about Eliza is how real it seems and how convincing it seems to be, given how simple it is.
MM: People absolutely, when they talk to it, they thought they were talking to a human or at least to something that understood them, and sometimes they would just tell it their deepest secrets, and they would ask other people to leave the room so they could have a confidential conversation with it. It was amazing
MB: Eliza’s creator, Joseph Weizenbaum, was actually pretty freaked out by the response he got. These were stock phrases, given automatically based on basic pattern matching. This is the same kind of technology that Microsoft Word uses to search and replace a phrase in a document.
You might tell Eliza you’re having a problem with your mother. Eliza doesn’t even know what a mother is. She just reaches into her robot brain and decides the most logical response, based on an algorithm.
But people were assuming Eliza understood them — and understood the context and emotion behind the things they were telling her. Weizenbaum hadn’t seen that coming.
MM: That really alarmed him, because he felt like that could be very dangerous to society, that people were would be taken in so easily by a completely unintelligent program.
MB: We’ve come a long way since Eliza. Ed says artificial intelligence has gotten a lot more… intelligent, if we want to go there. Especially over the last ten years.
EF: It’s been a real surprise. And the fact that these systems have advanced as far as they have as fast as they have has been one of the things that’s driven all of the interest in AI and the huge investments in industry.
MB: This AI boom was brought on by huge leaps in machine learning and neural networks.
[music]
EF: One of the early classic pieces of research for example was to build a system that could take a photograph and say, yes or no, is there a cat in this picture. Sort of a cat recognizer.
MB: Not all machine learning uses neural networks, but this cat recognizer did. Neural networks are inspired by the structure of the brain, connecting lots of tiny, simple units that work together to recognize patterns.
EF: And then you tell the machine, okay, figure out what cat-ness looks like.
MB: One part of the network would look at color, one part would look at ear shape, and so on.
EF: And then it will figure out a set of features or a procedure for taking some new photograph it’s never seen and figuring out what to do. Machine learning is basically building systems that can learn from examples or learn from experience. So that’s learning from examples.
MB: Machine learning can also work by trial and error. For example, if you want your AI to be able to operate a bumper car at a carnival.
EF: And the machine you tell it, okay, you have this thing, you can sort of turn clockwise or counterclockwise. You have these other two things on the bottom you can step on. And it just tries like a little kid. Right? Tries twisting the steering wheel and stepping on the pedals and seeing what happens and sort of figures out which things it can do to lead to good results.
MB: Machine learning is a big deal not just for natural language processing but for a huge chunk of AI. It turned out to be a really effective way to handle a lot of the problems computer scientists were grappling with.
EF: Things like detecting spam and fraud, planning routes and modeling traffic and weather, recognizing images, not to mention things like marketing and pricing.
MB: And, at the same time machine learning was leaping forward, we were making other big advancements, too. We got bigger and more powerful computers that could work with more sophisticated algorithms. And we got our hands on big data sets that these powerful computers and their algorithms could learn from, thanks in part to all the information on the internet.
EF: It’s those three things moving in sort of in sync that have led to the really rapid progress in recent years.
MB: And that progress… can’t be overstated. Melanie compares the difference between the Stu-bot program the postdocs created and modern language processing to the difference between the drip of a faucet and Niagara Falls.
[music]
EF: There were so many things that back then seemed like they were incredibly difficult — it would be decades or generations before computers would do them. And now we see computers starting to do those things. It really is a completely different world.
MB: We’re going to take a quick break, but when we come back, we’ll hear a modern language processor’s take on Ian Malcolm…
IAN MALCOLM FROM JURASSIC PARK: I’m simply saying that life… finds a way.
MB: and how it can be used for much more nefarious purposes. I’m Malcolm Burnley.
EF: And I’m Ed Felten.
MB: This is A.I. Nation.
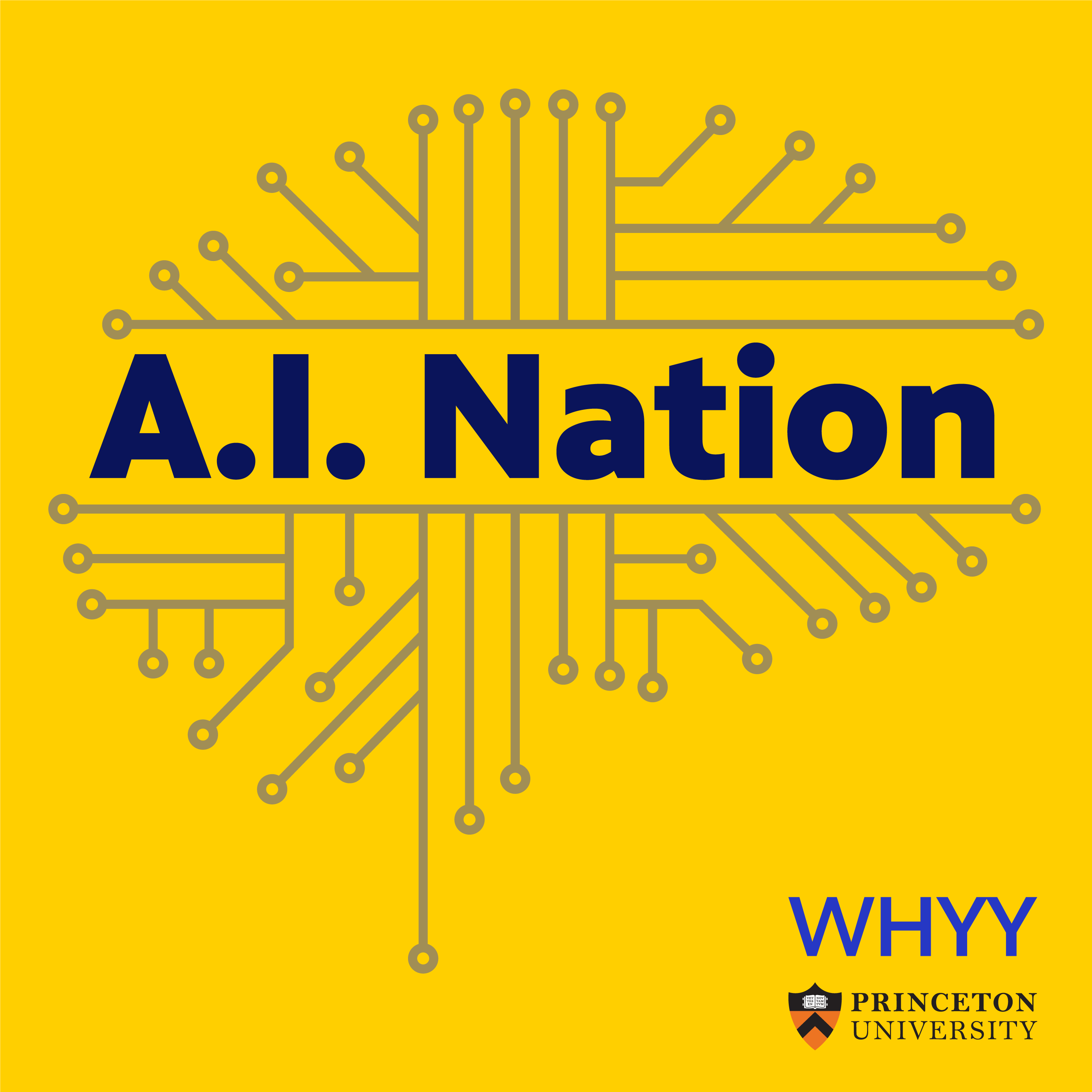
Listen to A.I. Nation
MALCOLM BURNLEY, HOST: Welcome back to A.I. Nation. I’m Malcolm Burnley.
ED FELTEN, HOST: And I’m Ed Felten.
[music]
MB: A lot of major tech companies, like Facebook and Google, are developing their own big natural language models. Melanie Mitchell, the researcher from the Santa Fe Institute, says they’re a foundational part of some of the things we use every day.
MM: Yeah in fact, Google web search, which all of us use all the time, is now partially powered by one of these large language models.
MB: Huh so is it when, if I start typing a word in my Google search, and Google completes the word, is that the language model?
MM: Well that’s one use of it, but also trying to figure out what your query is really trying to get at.
MB: One new, super advanced natural language model is called GPT-3. It was developed by OpenAI, a research lab founded by Elon Musk and funded by Microsoft.
One of the things that makes GPT-3 so advanced is its giant data set. To teach it how to best generate language, OpenAI fed it tons of examples: books, social media posts, all of Wikipedia.
MM: You know, people say that GPT-3 has memorized the entire internet, which I don’t know if that’s quite true, but it’s not far off.
MB: Another difference, and part of what makes today’s language models more advanced, is their ability to understand a larger context.
Ed: Or act like they understand, anyway.
MB: Stu-bot generated language on a word-by-word basis, which is part of the reason those short paper titles were able to sound convincing.
MM: It could have written a paper because you can generate as much text as you want with it. But it would have been completely incoherent because it was only using one word at a time to guess the next word.
MB: GPT-3, on the other hand, can work beyond this word by word relationship. It’s able to look at not just the last word it generated, but all the words it’s generated so far.
Scientists and AI enthusiasts have pushed it to do things Open AI never imagined. A comedian used it to write a short screenplay. A Silicon Valley product designer was even able to teach it how to code.
GPT-3 isn’t available to the public yet, so we reached out to OpenAI to see if we could get a demo or an interview. They said no.
But, the company had previously given access to a beta version of the AI to a limited group of researchers, including Melanie. And lucky for us, Melanie still had access.
MB: I would love if you don’t mind sharing your screen and we could kind of see what GPT-3 looks like.
MM: OK, let me try and do that here.
MB: My producer and I watched over Zoom as Melanie pulled open the program on her computer.
MB: So maybe you could describe a little bit of what we’re looking at.
MM: So this is a web, a web page that gives the interface it. It’s called Playground, and it has a text entry box and a submit button.
MB: The way GPT-3 works is that you give it a text prompt — a sentence, a paragraph — and it can use everything it’s learned from, you know, memorizing the entire internet, to continue that prompt for you.
In honor of the nerdy postdocs, we decided to ask GPT-3 to create something that might have been written by Ian Malcolm. We gave it the text of one of his famous monologues. It’s from the scene in Jurassic Park where he’s sitting around a table with the rest of the main cast: there’s Ellie Sattler (played by Laura Dern), her boyfriend and fellow scientist, the owner of the park, and a lawyer who represents the board members. The owner and the lawyer are excited, talking about how much money they’re going to make on all these dinosaurs they’ve created. Ian Malcolm is… not on board.
IAN MALCOLM FROM JURASSIC PARK: I’ll tell you the problem with the scientific power that you’re using here. It didn’t require any discipline to attain it. You know, you read what others had done, and you took the next step. You didn’t earn the knowledge for yourselves, so you don’t take any responsibility for it. You stood on the shoulders of geniuses to accomplish something as fast as you could and before you even knew what you had, you patented it, and packaged it, and slapped it on a plastic lunchbox and now you’re selling it! Your scientists were so preoccupied with whether or not they could they didn’t stop to think if they should.
MM: and now I’m going to submit it and have GPT-3 complete it.
MB: Wow. Wow, so for the listener, a bunch of text just appears on the screen, pretty instantaneously but one line at a time. And it looks like it’s done already.
MM: Okay so here’s what GPT-3 generated.
Ladies and Gentleman, computer scientist Melanie Mitchell as computer-generated Ian Malcolm.
MM: Well, there’s a name for this. It’s called plagiarism, but you don’t even care because you don’t even know what you don’t know. So while you’re blithely disrupting (and it misspells that) what you do and do not understand, I’ll be busy doing something.
[MB laughs]
MB: That response cracked me up because it so thoroughly captured Ian Malcolm’s attitude. And Melanie pointed out that, while GPT-3 hasn’t exactly gotten the content right, it picked up on something.
MELANIE MITCHELL AND IAN MALCOLM, TOGETHER: You stood on the shoulders of geniuses to accomplish something as fast as you could.
MM: So this is, that’s sort of vaguely like plagiarism? It’s not exactly. But somehow the machine has associated it with plagiarism, which is, you know, it sort of sounds like it’s understanding, in a way, what the what the paragraph was about.
EF: But the content’s a little bit off, right? It doesn’t quite follow from what he said before. I mean it’s him, but not quite.
MB: We ran Ian Malcolm’s speech through a few more times, in a few different ways. We gave GPT-3 a longer response length.
MM: Let’s try this.
MB: Alright. Hm. Oh that’s an interesting response.
MM: It says: It is the responsibility of the people who are doing this work to follow the examples of the great men and women of science. In the book Cosmos, Carl Sagan quoted the sentiment of the unnamed Nobel Laureate, and added the following.
MB: Sounds pretty legit. Except…
MM: Colon, in the book Cosmos, Carl Sagan also wrote colon Sagan also wrote colon…
[Both laugh.]
MM: Getting in this repetitive loop here.
MB: GPT-3 isn’t perfect… Sometimes it sounds really convincing, and other times it starts convincingly but then toddles off into nonsense. The longer the text you ask it to generate, the more likely it is this will happen.
But other strange things happen, too. At one point, we typed “Ian Malcolm” and then a colon before the speech, telling GPT-3 who was speaking.
MB: Yeah, could you read this part?
MM: Okay. It says, Ian Malcolm colon. We’ve always been pawns, Mr. Hammond. It was inevitable. Then it says Dr. Ian Malcolm, to Ellie: I’m sorry I’ve been impolite. I haven’t even asked you, What brings you here? Ellie Sattler: Dinosaur eggs. Dr. Ian Malcolm: Dinosaurs. Ellie Sattler: We found them in Montana. Dr. Ian Malcolm: And you brought them back. Ellie Sattler: Actually, we were about to destroy them when the power went out.
EF: Now that’s kind of amazing. You gave it Ian Malcolm colon and it figured out to write what could really be a scene from one of those movies. It’s got the right characters in it. You didn’t tell it Ellie Sattler goes with Ian Malcolm. You didn’t tell it that there’s a thing called a script where there’s a name and then a colon, and then something that that person would have said. You see the tone or attitude switches from one character to the other, and it says something like Malcolm to Ellie, again, a thing you’d see in a script. It just kind of absorbed the ability to pretend to be them, or to pretend to write a script about them, out of the huge amount of information that it started with.
MB: Right it’s pretty fascinating. Because it’s clearly in the world of Jurassic Park. I had to do a fact check to make sure that this language wasn’t actually in the movie.
EF: And it’s not?
MB: And it’s not. There are little bits and pieces, like the power went out at one point in Jurassic Park, so that’s also from the movie, as Ellie Sattler (GPT-3 Ellie Sattler) says, but it’s kind of mish-mashing all of these things that are from the movie into a dialogue that never happened.
EF: Yeah it’s kind of amazing how well it’s able to fake this. So sometimes [GPT-3] gets it just right and kind of gets it just right like this. And sometimes it doesn’t.
MB: Yeah and if you try a few times, you’ll probably get it, and you want to cherry pick, it can seem so astounding in terms of its accuracy because it can do that. But at the same time it might be one out of four, one out of five.
EF: Which is still pretty incredible, right, that it’s able to do it at all. So going forward I think you’re going to see the future GPT-3-like systems that get it wrong less often and get it just right more often. But you can see here that there’s a sense where the machine seems almost creative.
MB: This still doesn’t necessarily mean that GPT-3 can understand the language it takes in, or the language it generates. As much as we anthropomorphize technology, it is no closer to “thinking” like us than Eliza is.
MM: It absolutely is not like a person. When I’m reading that paragraph, for instance, the paragraph about Ian Malcolm, I know what it means to slap something on a lunch box. I know what standing on the shoulders of somebody means. I know because I’m mapping it on, all onto mental models of the world, whereas GPT-3 doesn’t have anything like that. It doesn’t know what a lunchbox is, it doesn’t know what standing on shoulders is, it doesn’t know even what shoulders are.
MB: All GPT-3 has is the text it’s digested, and a neural network model of probabilities — which lets it simulate us. But it is not us.
MM: There was a recent paper that referred to these language models as stochastic parrots. [laughs] So that means that basically a parrot will memorize something that a humans said and parrot it back. And a stochastic parrot is a parrot that sort of randomly goes from parodying one thing to another thing to another thing —
MB: –smashing all those bits together. A parrot doesn’t sound particularly frightening or dystopian.
EF: It depends how clever the parrot is. I think I’ve seen that movie.
[music]
MB: But, just like with Eliza, GPT-3’s creators have raised some red flags. When OpenAI announced the previous version, GPT-2, just two years ago, they said it was too dangerous to release to the public in full. Ed, do you think a technology like GPT-3 could be dangerous?
EF: There certainly are some risks that would come with it. One of them is that it would get more difficult to tell whether you’re communicating with the person.
MB: It could, possibly, be used for disinformation. Melanie wanted to check this out on GPT-2 a couple of years ago.
MM: I gave it a prompt: “Vaccines have been shown to cause autism.” Which is obviously a false statement. But I wanted to see what its capability was for this, for generating disinformation.
MB: And, low and behold, GPT-2 generated a paragraph, full of falsehoods about vaccines, that sounded like a real person had written it. It was authoritative. It even gave citations with web links to scientific papers — though the scientific papers were totally unrelated.
Melanie discovered GPT-2 can spread lies.
If we’re not careful, we run the risk of making AI just like us, warts and all. Instead of creating computer brains that are unbiased and unemotional, they’ll be the opposite. It’ll be human in all the wrong ways.
MM: One thing that a lot of people are talking about now are the subtle biases that these language models learn by being trained on human text. So they can be very racist and sexist in the texts that they generate.
MB: For example, somebody else tried typing in “Two muslims went into a bar,” and found that the AI often finished the story by making them terrorists.
EF: AI is a thing built by humans. We make the decisions about how to apply it and where to apply it. The development of AI is driven by commercial considerations, and so that ties into how our economy works and what it rewards and so on. Absolutely, the development and use of A.I. reflects the best and worst of our society in a lot of ways.
[music]
MB: We’ll talk about those ways, including how human bias gets into AI and what we can do about it. Later on, in A.I. Nation.
In our next episode, we’ll take you for a ride in a self driving car, and learn a bit about why engineering them is a harder problem than we originally thought. We’ll also hear from a former Google engineer, who quit her job rather than work on AI she said could lead to killer robots. (It turns out… we should be kind of worried about killer robots.)
At each step along the way, we’re going to ask big questions about the drawbacks and black boxes that AI can create. We’ll be probing if the risks outweigh the rewards and even whether humans should be using AI in some places at all.
EF: One of the things we’ve learned in recent years is how much is at stake in decisions about AI, about how it’s developed and how it’s deployed, how it’s used, and the ways in which AI systems can have really negative effects, can raise risks or can magnify discrimination or injustice, unless we take care to keep that from happening.
This is a moment when AI is becoming widespread in the systems that we interact with all the time.You’re seeing issues related to AI being talked about in Washington. You’re seeing people really starting to debate what is the role of technology in our lives.
MB: Subscribe to A.I. Nation so you don’t miss an episode. You can find us wherever you get your podcasts.
A.I. Nation is produced by me, Malcolm Burnley and Alex Stern. My co-host is Ed Felten. Thanks, Ed!
EF: Sure thing, Malcolm!
MB: We have editing help from Katie Colaneri and John Sheehan. This podcast is a production of WHYY and Princeton University.
[music fades]